Text Analysis in Healthcare Feedback Surveys
Text analysis is a powerful tool that can transform unstructured patient feedback into actionable insights. It involves analyzing patient comments, suggestions, or complaints to identify recurring themes, sentiments, and areas for improvement.
Essentially, you’re turning unstructured data into actionable insights — with some steps to follow in between. Let’s see how this works in practice (pun intended).
What is text analysis?
Text analysis refers to the process of systematically reviewing and interpreting written responses to uncover patterns, trends, and sentiments.
In healthcare, this means going through qualitative feedback — such as open-ended survey responses — to identify key topics like patient satisfaction, communication quality, or areas of concern.
Doing this requires using tools like keyword extraction, sentiment analysis, and topic modeling. Here is an example of sentiment analysis in action:
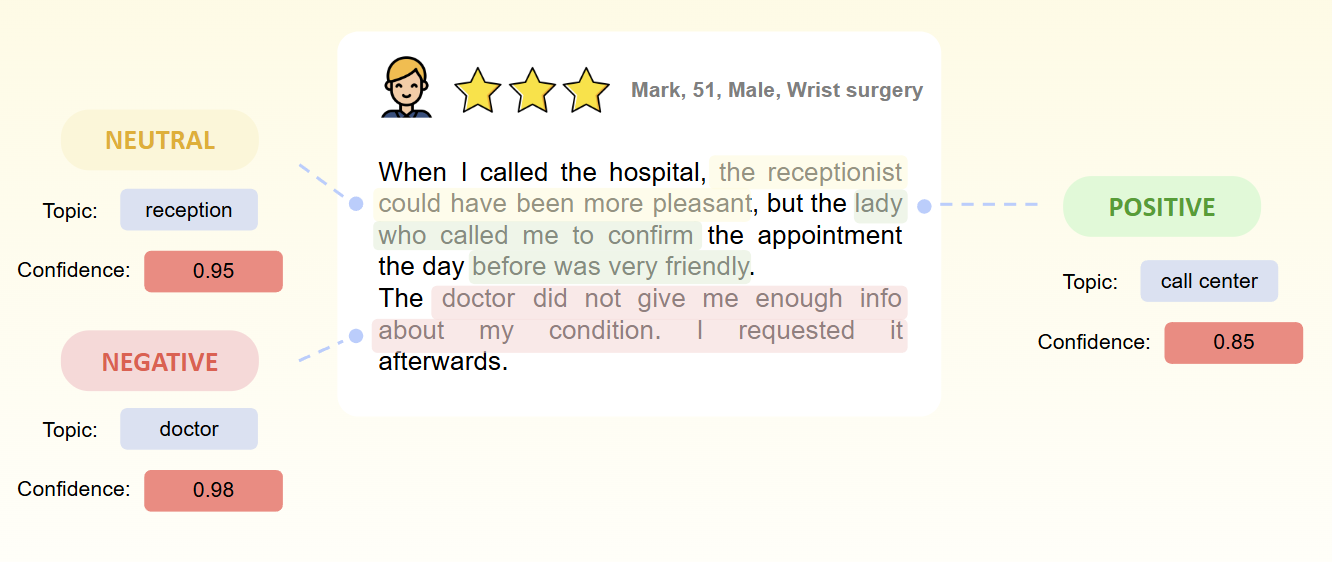
The topic/sentiment analysis tool is also very industry-specific. To be certain of your outcome, use a tool that is trained for the healthcare space. Similarly, if you'd like to develop your own plug-in for this, make sure you have the required expertise in both healthcare and development tools.
Prerequisites for effective text analysis
Before diving into text analysis, it’s crucial to ensure the process is set up for success. Here are some key prerequisites to consider:
- High-quality data collection: Ensure that open-ended questions are clear and encourage meaningful feedback. A well-designed survey elicits responses that are both relevant and detailed, improving the quality of your text data.
- Selecting the right text analysis tools: Use tools tailored to the specific needs of healthcare feedback. NLP (Natural Language Processing) software, sentiment analysis, and keyword extraction platforms should be chosen based on their ability to handle medical terminology and patient-centric language.
- Comprehensive text preprocessing: Preprocess your text data by cleaning it (removing irrelevant terms, handling spelling errors, and normalizing data) to ensure consistent and accurate results. This step is crucial for improving the precision of the analysis.
- Defining clear goals for analysis: Establish clear objectives before beginning your analysis. Are you looking to measure patient satisfaction, detect areas for improvement, or analyze specific aspects of patient care? Defining your goals will help guide the analysis process and ensure relevant outcomes.
- Integration with quantitative data: Combining text analysis with quantitative survey data can provide deeper insights. Use text responses to explain trends observed in quantitative metrics like Net Promoter Scores (NPS) or satisfaction ratings, leading to a more holistic view of patient feedback.
- Ongoing updates to models and algorithms: Healthcare feedback evolves over time, as does the language patients use. Regularly updating your text analysis models ensures that you capture the latest trends, address new issues, and improve the accuracy of insights over time.
- Tailoring analysis to the healthcare sector: Generic NLP models might not adequately process healthcare-specific terminology or nuanced patient feedback. Using models that are designed to understand the emotional and medical context of healthcare will improve analysis accuracy.
Common mistakes in text analysis
Even with the right tools and processes in place, organizations often make mistakes when conducting text analysis. Here are some of the most common pitfalls to avoid:
- Over-reliance on automation: Fully automated analysis might miss nuances, context, or emotional cues that require human interpretation.
- Ignoring the context of responses: Analyzing feedback without considering factors like the type of treatment or patient demographics can lead to surface-level insights.
- Misinterpreting sentiment scores: Sentiment analysis tools can misread sarcasm or complex emotions, leading to inaccurate conclusions.
- Overlooking rare but critical feedback: Focusing only on common themes might cause you to miss less frequent but crucial issues, such as safety concerns or treatment delays.
- Big-data paralysis: Gathering huge volumes of data is great, but it comes with the risk of simply not knowing what to do (or how to do something) with these huge quantities of data.
The takeaway
Effective text analysis in healthcare surveys requires thoughtful preparation and ongoing refinement. However, once you set it up, it quickly becomes an invaluable tool for uncovering the deeper meanings behind patient feedback in healthcare.
Start your free pilot project today
Analyze patient feedback. Optimize workflows to deliver a superb patient experience. Stop your never-ending battle with patient retention.